How Big Data Can Help Feed Us
Artificial Intelligence will integrate and analyze diverse data and models to make farming recommendations for more bountiful harvests in Ethiopia
By Marc Ballon
(Illustration/Raymond Biesinger)
An Ethiopian farmer plants teff, a grain used in traditional flatbread, as she has done for years. However, a short rainy season wipes out most of her crop, threatening her and other farmers’ livelihoods and food security.
Now, imagine that same farmer discovers beforehand that this season will be particularly dry. She learns how long crops will take to grow given the anticipated weather trends and which ones might thrive in such conditions. Instead of teff, she grows fast-maturing mung beans and has a decent harvest.
Thanks to Yolanda Gil, a USC Viterbi School of Engineering professor and one of the world’s foremost artificial intelligence (AI) experts, such a scenario could one day become a reality.
Three years ago, Gil and her collaborators visited Ethiopia intending to develop an AI platform that could make recommendations to policymakers to increase food production and reduce the likelihood of famine.
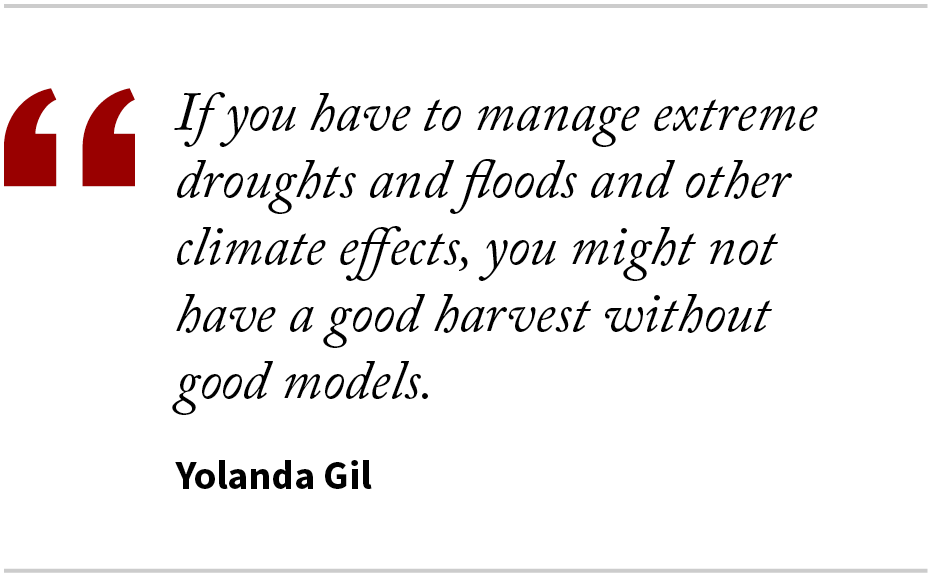
“If you have to manage extreme droughts and floods and other climate effects, you might not have a good harvest without good models,” says Gil, director of New Initiatives in AI and Data Science at USC Viterbi and former president of the Association for the Advancement of Artificial Intelligence (AAAI).
Gil knew she was on to something when she met with a high-level official from the Ministry of Water and Energy. He pointed out that the agricultural, water and other reports covering his desk were each narrowly focused. Worse, some of the recommendations contradicted each other. What they needed was an integrated view of the effects of climate on agriculture.
By contrast, Gil is developing a novel system that uses AI to integrate models and provide a comprehensive roadmap for decision-makers to help significantly boost Ethiopia’s agricultural output.
The Model INTegration framework, or MINT, employs AI to integrate heterogeneous models from separate disciplines efficiently and quickly. MINT currently includes climate, hydrology and agriculture models for different areas of Ethiopia to understand how government interventions (such as subsidies) can mitigate the effects of droughts and floods.
“We have become really good at running single models to generate reports with recommendations looking at only a single slice of the problem,” Gil says. “But it’s very challenging to put together comprehensive models across disciplines, across processes. That’s what my AI research is about.”
Feeding Ethiopia
Agriculture is the backbone of the Ethiopian economy. Mixed farming, which includes growing crops and raising livestock, accounts for nearly 40% of the country’s gross domestic product and more than 70% of the workforce, according to the United States Agency for International Development. Teff, wheat, maize, sorghum and barley, among other crops, make up the core of the food economy.
Even in the best of times, feeding the country’s roughly 115 million people can be challenging.
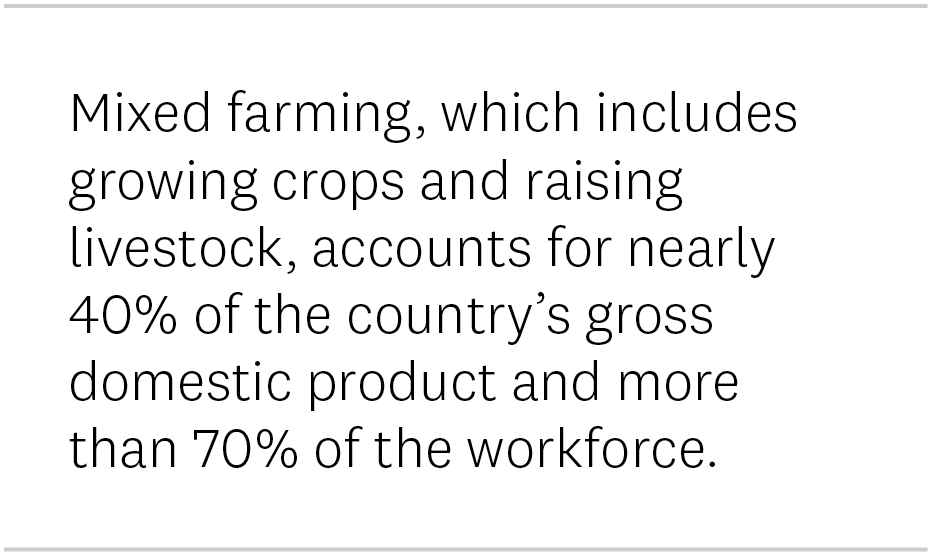
Bordering two distinctly different climate regimes — one wet and one dry — Ethiopia and other countries in sub-Saharan Africa experience periods of exceptionally low rainfall, says Lowell Stott, a professor of earth sciences at the USC Dornsife College of Letters, Arts and Sciences.
“The eastern part of the Nile Basin is highly vulnerable to droughts,” adds Essam Heggy, a USC Viterbi expert on water evolution in Earth’s arid environments.
Indeed, Ethiopia’s lowland areas are enduring one of the most severe dry periods in 40 years. “The prolonged drought is compromising fragile livelihoods heavily reliant on livestock and deepening food insecurity and malnutrition,” according to the United Nations Office for the Coordination of Humanitarian Affairs.
Ethiopia also suffers from occasional flooding that can ruin crops, giving farmers more to worry about.
Still, many believe the country’s farming sector might have a bright future, the challenges notwithstanding.
Ethiopia’s different climate and soil types make it possible to grow diverse crops, says Gebissa Yigezu of Haramaya University in Ethiopia. Additional government incentives, improved infrastructure and increased credit and donations from international organizations might spur higher crop yields and exports, he explains in the academic paper “The Challenges and Prospects of Ethiopian Agriculture.”
MINT’s integrated modeling aims to make those efforts much more effective.
MINT in action
With a grant from the Defense Advanced Research Projects Agency, Gil began working on MINT in 2019. She finished a prototype one year later. Gil says MINT represents an advance in artificial intelligence. “Goal-oriented problem solving is not new in AI. But goal-oriented problem solving where you are integrating models from different scientific disciplines is a completely new approach.”
In creating MINT, which spans AI research in knowledge representation, reasoning and machine learning, Gil first converted diverse data for weather, soil, topography and many others into data that computer modeling could understand and use.
Then she provided the advanced AI system with data and models from different disciplines and goals inspired by questions from scientists such as: What crops would give a higher yield next year? When should the planting of maize start so it can be harvested before flooding starts? Should the government subsidize fertilizers?
After receiving the knowledge from scientists about how their models work, Gil says, MINT aggregates the data and analyzes various possible future scenarios. “With MINT, the AI could do most of the work so that we can come up with answers in a couple of weeks instead of a couple of years,” she says.
Gil says a rudimentary version of MINT could demonstrate how AI integrates models to make more effective recommendations for decision-makers.
With collaborator Belete Berhanu, a hydrologist at Addis Ababa University, Gil co-authored a paper last year that appeared at the First International Workshop on Social Impact of AI for Africa held at the annual AAAI conference. Gil’s team has been generating thousands of model runs under different initial conditions to explore different assumptions and scenarios.
When operational, MINT “could help in boosting crop production because it provides the right information at the right time” for different types of farming, says Yihenew Zewdie, an Ethiopia-based expert in food systems and natural resource governance.
Solving other intractable problems
With different data and simulation models, MINT could play an important role in addressing other significant problems ranging from fires to floods and other natural disasters.
The MINT modeling framework is being used in a research project headed by the University of California, San Diego’s San Diego Supercomputer Center to bring the power of AI to help firefighters strategize how best to plan controlled burns and manage unexpected blazes.
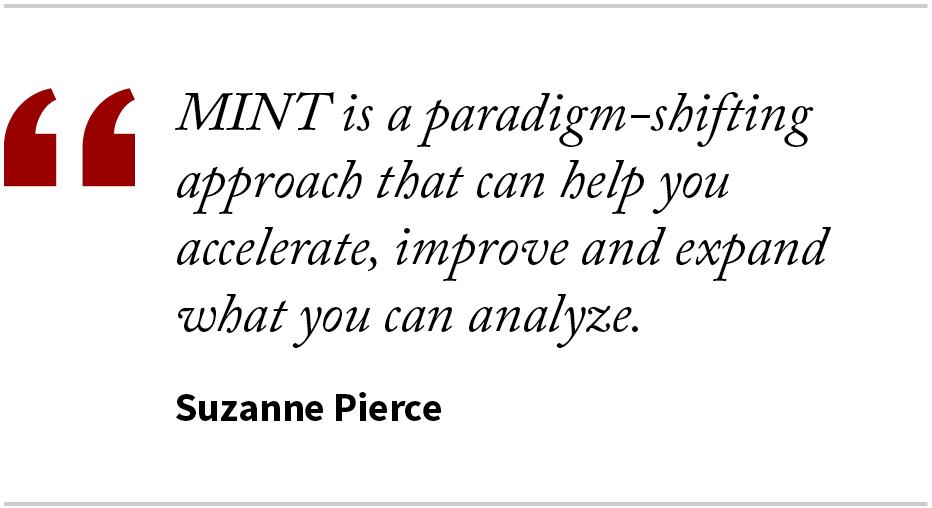
Gil also works with the Technical University of Munich to model the impact on streamflow and farming from changes in the timing and size of snowmelt in central Kyrgyzstan.
In a collaboration with the Texas Advanced Computing Center at the University of Texas at Austin, Gil is working with models of groundwater that help predict water availability in Texas aquifers under different conditions.
Gil works with Suzanne Pierce and others in the Texas Disaster Information System (TDIS), which serves as an information clearinghouse to support improved natural disaster planning, response, recovery and mitigation efforts in Texas. Pierce leverages MINT and its AI technology for floods to predict and identify the most at-risk areas.
“MINT is a paradigm-shifting approach that can help you accelerate, improve and expand what you can
analyze,” Pierce says. “I think it can make a world of difference across the many wicked problems that society is currently facing.”